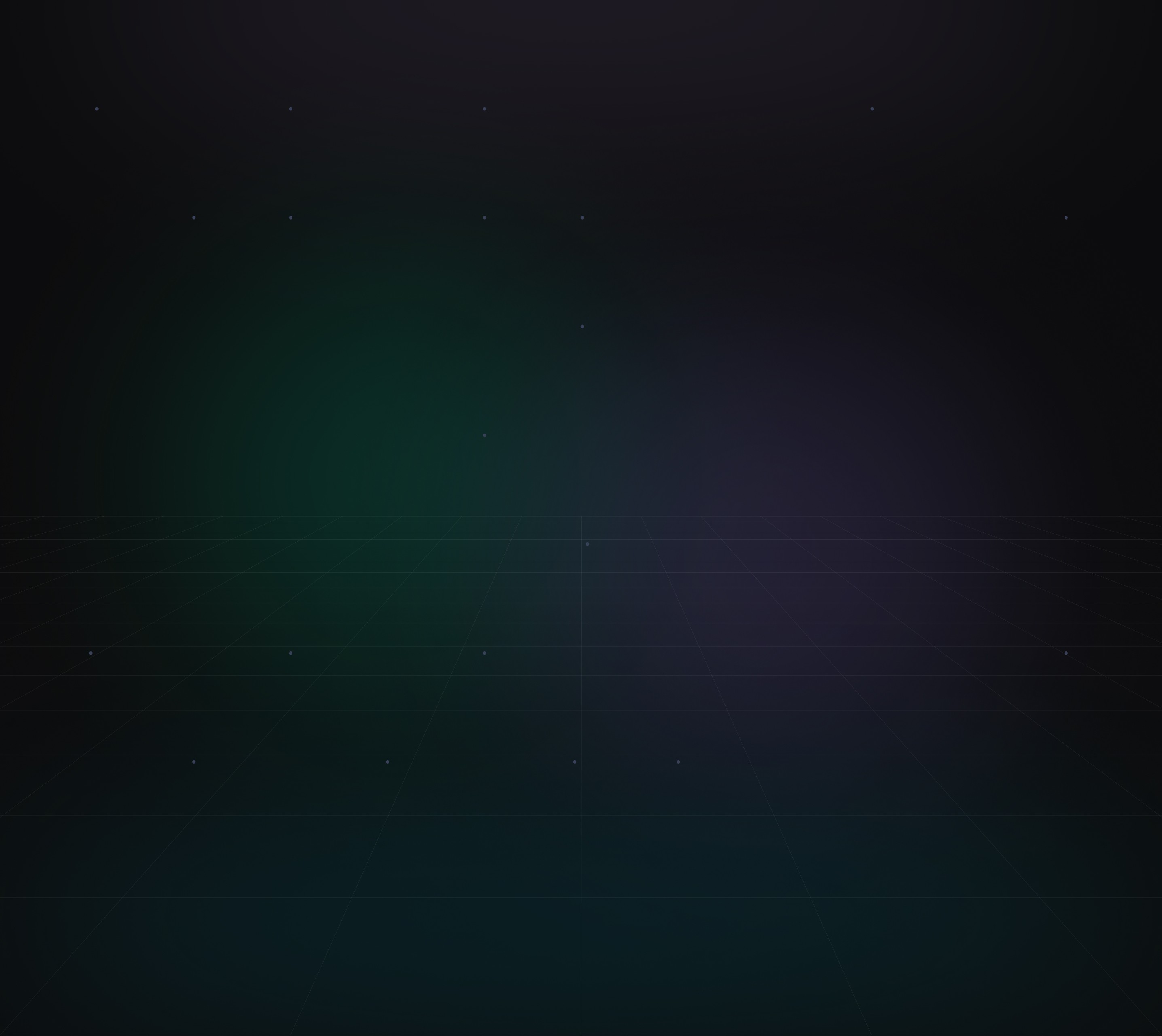

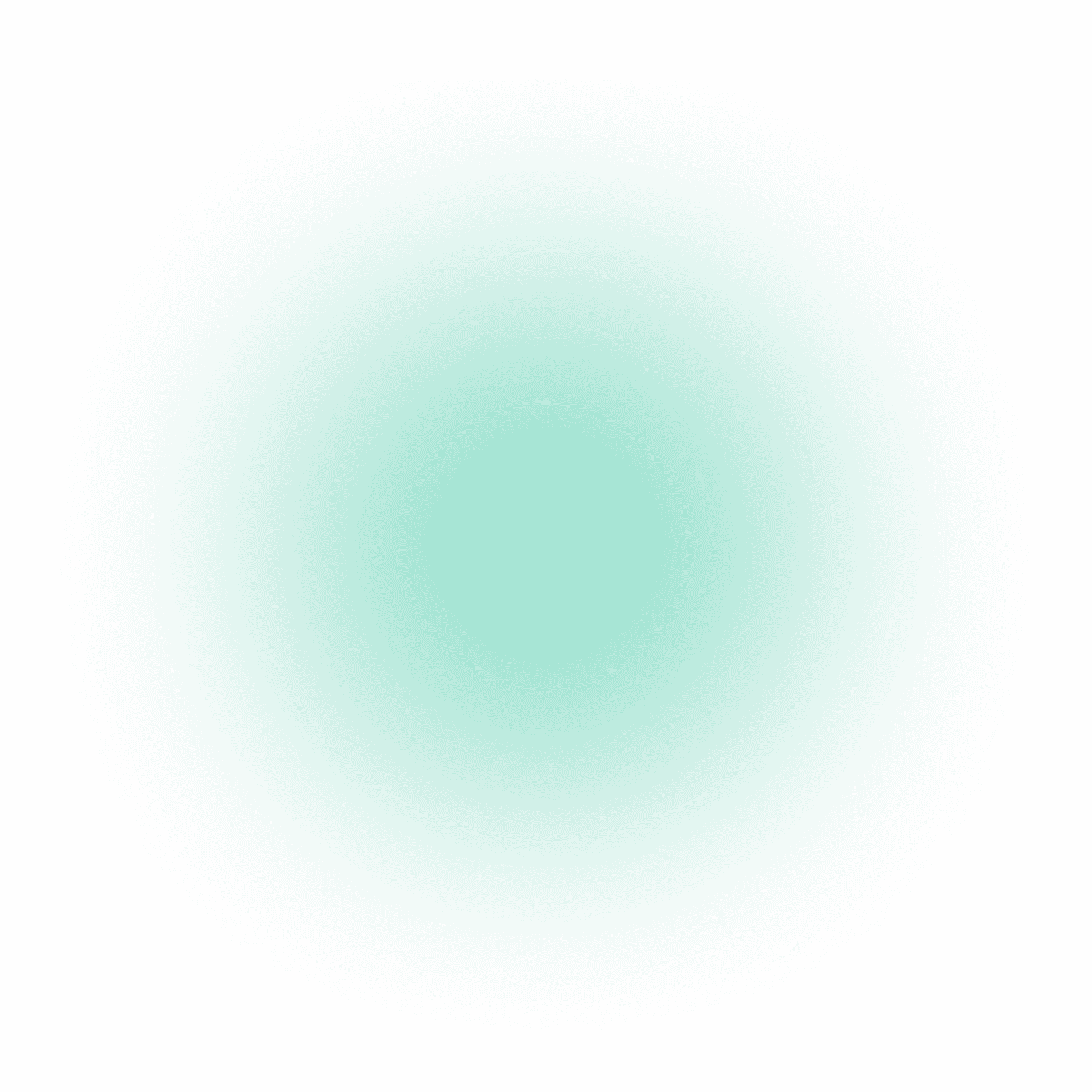

The Relationship Between Agentic LLMs and Systems Engineering: A Lightbulb Moment for Everyone
Discover the fascinating relationship between agentic Large Language Models (LLMs) and systems engineering in a way anyone can understand.
Author
D Team
Aug 31, 2024
The Relationship Between Agentic LLMs and Systems Engineering: A Lightbulb Moment for Everyone
Artificial Intelligence (AI) is transforming many fields, and one of its most exciting developments is the emergence of agentic Large Language Models (LLMs). Meanwhile, systems engineering has long been a method for designing, integrating, and managing complex systems throughout their lifecycle. But what happens when we combine these two concepts? Understanding this relationship can spark a "lightbulb moment" of clarity for everyone—even those without a technical background.
Using the Feynman Technique, a powerful learning method that involves breaking down complex ideas into simple, understandable terms, we’ll explore how agentic LLMs intersect with systems engineering and why it matters.
1. What is an Agentic LLM? Let's Simplify
To understand the relationship between agentic LLMs and systems engineering, we need to first clarify what these terms mean:
Large Language Models (LLMs): LLMs like GPT-4 are AI systems trained to understand and generate human-like text. They predict the next word in a sentence based on vast amounts of data, enabling them to perform tasks like answering questions, summarizing information, and even engaging in conversations.
Agentic LLMs: When we talk about agentic LLMs, we are referring to LLMs that have been designed to act as agents—autonomous entities that can perform tasks, make decisions, and interact with their environment based on predefined goals. Unlike traditional LLMs that merely generate text, agentic LLMs can initiate actions, seek additional information, and learn from their interactions, making them more dynamic and adaptive.
Think of an agentic LLM as a smart assistant that not only answers questions but also takes actions to help achieve specific objectives, like a digital assistant that can book your flights, manage your schedule, or troubleshoot issues by itself.
2. What is Systems Engineering? A Simple Breakdown
Now, let’s break down systems engineering into simple terms:
Systems Engineering: This is a multidisciplinary approach used to design, develop, and manage complex systems. A system could be anything from a car or a software application to a spacecraft or a smart city. Systems engineering focuses on ensuring that all parts of the system work well together and meet the desired requirements.
Think of systems engineering as constructing a building. It involves designing the foundation, framework, electrical systems, plumbing, and finishing—all while ensuring they work together harmoniously to create a safe and functional structure.
3. How Do Agentic LLMs and Systems Engineering Interact?
Now that we’ve defined the two concepts, let’s explore how they interact using the Feynman Technique:
Imagine an Intelligent, Self-Improving System: Consider a smart city, which is a complex system that involves traffic management, public safety, energy distribution, and more. A systems engineer designs these components to work together efficiently. However, what if the system could learn, adapt, and optimize itself in real-time? This is where agentic LLMs come in.
Agentic LLMs as Autonomous System Managers: In a smart city, an agentic LLM could act as a central control agent. It could analyze traffic patterns and adjust signal timings to reduce congestion, predict energy consumption peaks to optimize grid loads, or even respond to emergency situations by coordinating with different city departments—all autonomously.
Dynamic Decision-Making and Adaptation: Unlike traditional systems that rely on pre-programmed rules, an agentic LLM in systems engineering can dynamically make decisions based on real-time data. It can also learn from past actions and outcomes, becoming smarter and more efficient over time. This adaptability is crucial for complex systems where variables can change unpredictably.
4. Why is This Relationship a "Lightbulb Moment"?
Understanding the synergy between agentic LLMs and systems engineering can change how we think about designing and managing complex systems:
From Static to Dynamic Systems: Traditionally, systems are built to function according to predefined rules. However, incorporating agentic LLMs transforms these systems into dynamic, self-optimizing entities. It’s like moving from a fixed, one-size-fits-all solution to a custom-fit, evolving approach.
Enhanced Problem-Solving: With agentic LLMs, systems gain a layer of intelligence that enables them to solve problems proactively rather than reactively. Imagine a smart grid that can predict a power outage and reroute energy supply before it happens—this is made possible by combining agentic LLMs with systems engineering principles.
Greater Efficiency and Innovation: This relationship opens up possibilities for creating more efficient, resilient, and innovative systems across various fields, from healthcare and transportation to finance and manufacturing.
5. Using the Feynman Technique to Understand the Integration
Applying the Feynman Technique further, let's distill this concept into an analogy:
Analogy: Imagine a team of construction workers building a bridge (representing systems engineering). Normally, they follow a blueprint and work according to strict instructions. Now, imagine one of these workers is an AI assistant who can think, learn, and suggest improvements. This worker notices that using a different material could make the bridge both cheaper and stronger. It dynamically adapts the blueprint and optimizes the workflow, all while collaborating with the rest of the team. The AI assistant represents the agentic LLM, enhancing the system (bridge building) with real-time intelligence and adaptability.
6. Real-World Applications: Where This Relationship is Already Making an Impact
The combination of agentic LLMs and systems engineering isn’t just theoretical; it’s already being applied in several fields:
Autonomous Vehicles: Agentic LLMs help in managing complex systems of self-driving cars by processing vast amounts of data from sensors, making decisions in real-time, and learning from each driving experience.
Smart Manufacturing: Factories use agentic LLMs to optimize production lines, predict equipment failures, and manage supply chains dynamically.
Healthcare Systems: In hospitals, agentic LLMs can manage patient flows, predict demand for resources like ICU beds or medications, and assist in diagnostic decision-making by learning from vast datasets.
7. Conclusion: A New Era of Intelligent Systems
By combining agentic LLMs with systems engineering, we are moving toward a new era of intelligent, self-optimizing systems. This integration offers tremendous potential for improving efficiency, innovation, and problem-solving capabilities across various industries. Understanding this relationship can indeed be a "lightbulb moment," illuminating the path to a more advanced and adaptive technological future.
With the Feynman Technique as our guide, even complex ideas like these can become clear and approachable, sparking curiosity and understanding in everyone, regardless of their technical background.