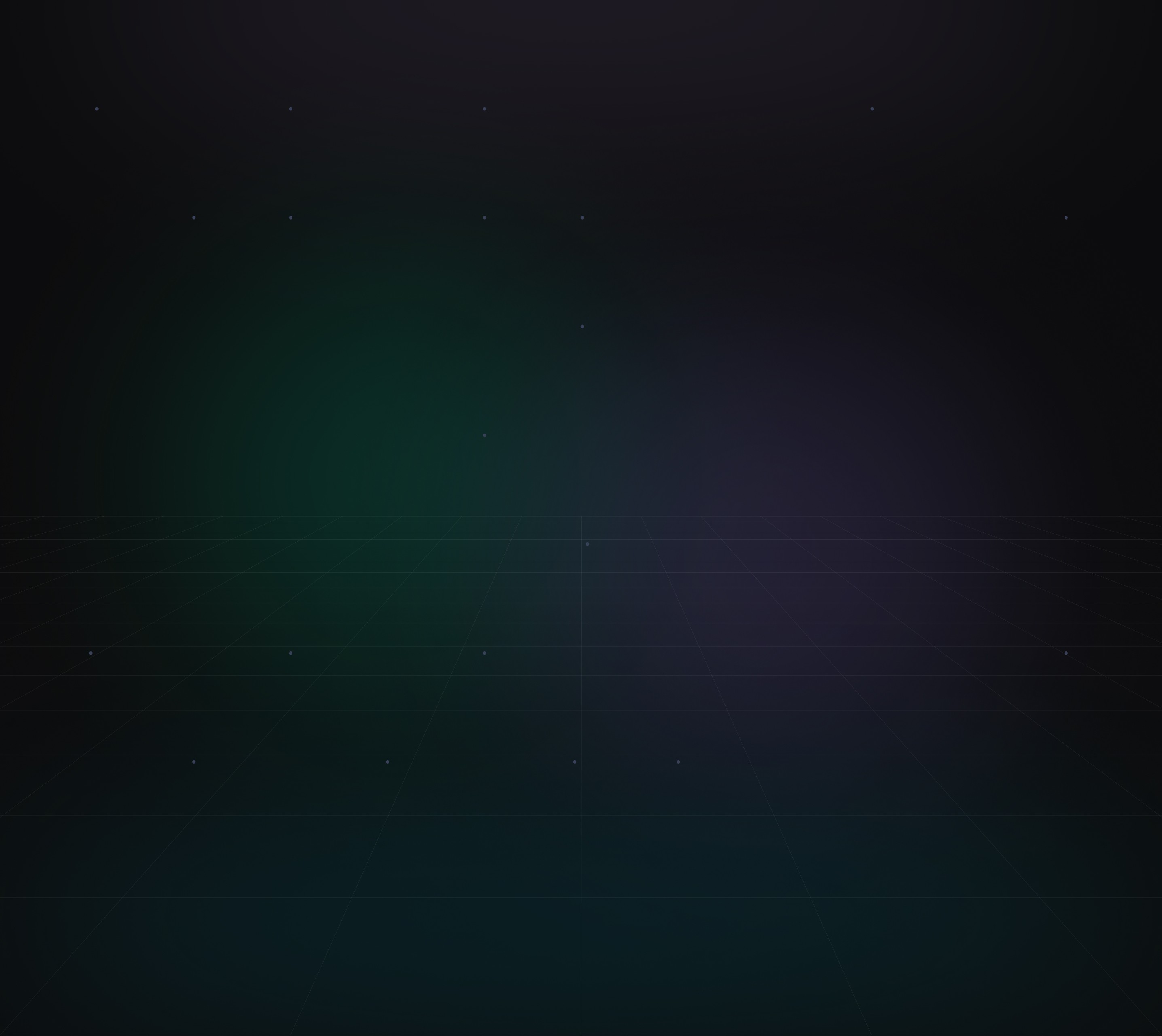

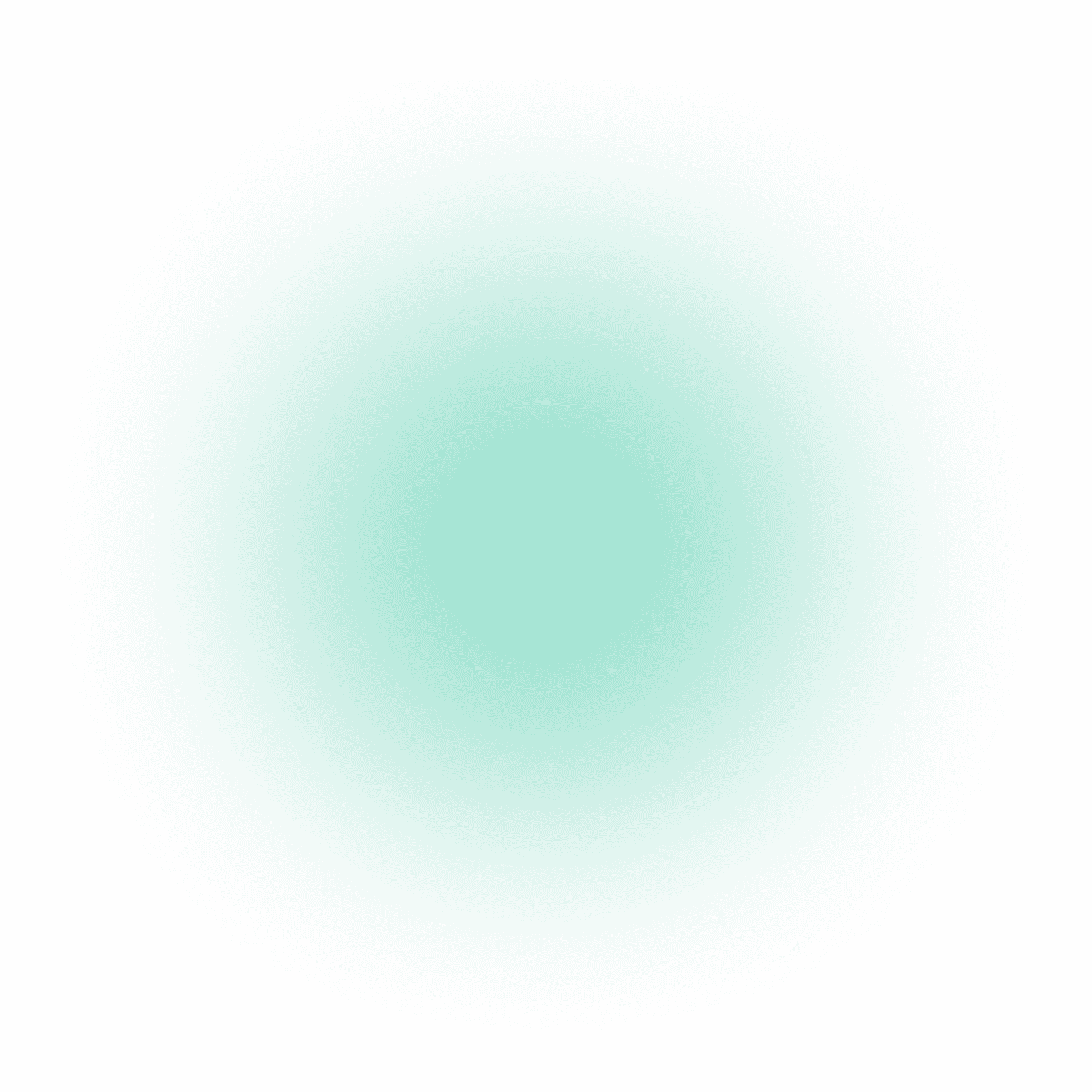

How Large Language Models Are Changing the Way We Search for Informatio
Discover how Large Language Models (LLMs) are impacting the way we search for information. This article explains how LLMs are transforming search experiences with AI-powered answers, enhanced relevance, and conversational capabilities.
Author
D Team
Aug 31, 2024
How Large Language Models Are Changing the Way We Search for Information
Searching for information online has become second nature to most of us. For decades, search engines like Google, Bing, and Yahoo have been the primary tools for finding answers, learning new things, and exploring the web. However, the advent of Large Language Models (LLMs) like GPT-4 is changing how these search engines operate and how users interact with information. This article explores the impact of LLMs on search engines, tracing the evolution from keyword-based searches to AI-driven, conversational experiences.
1. The Evolution of Search Engines: A Brief History
To understand how LLMs are reshaping search, let’s first take a quick look at the evolution of search engines:
1990s - Early Search Engines and Keyword Matching: In the early days of the internet, search engines like Archie, AltaVista, and Yahoo relied on simple keyword matching to find relevant web pages. They were basic but revolutionary tools that indexed websites and matched them with user queries based on keywords.
2000s - Google's Rise and PageRank Algorithm: The launch of Google in 1998 marked a significant shift. Google introduced the PageRank algorithm, which ranked web pages based on the number and quality of links pointing to them. This made search results more relevant and reliable. Google’s simple yet effective interface and superior relevance helped it dominate the search market.
2010s - Semantic Search and Machine Learning: By the 2010s, search engines began incorporating machine learning and semantic search techniques. Google’s Hummingbird (2013) and RankBrain (2015) updates were major milestones. RankBrain, in particular, was a machine-learning-based algorithm that could understand the meaning behind queries, not just the exact words. This allowed for more contextually relevant results.
2020s - The Rise of LLMs and AI-Powered Search: The launch of powerful LLMs like GPT-3 (2020) and GPT-4 (2023) marked the beginning of a new era in information retrieval. These models have the capability to understand and generate human-like text, offering more accurate, nuanced, and conversational responses to user queries.
2. The Role of LLMs in Modern Search Engines
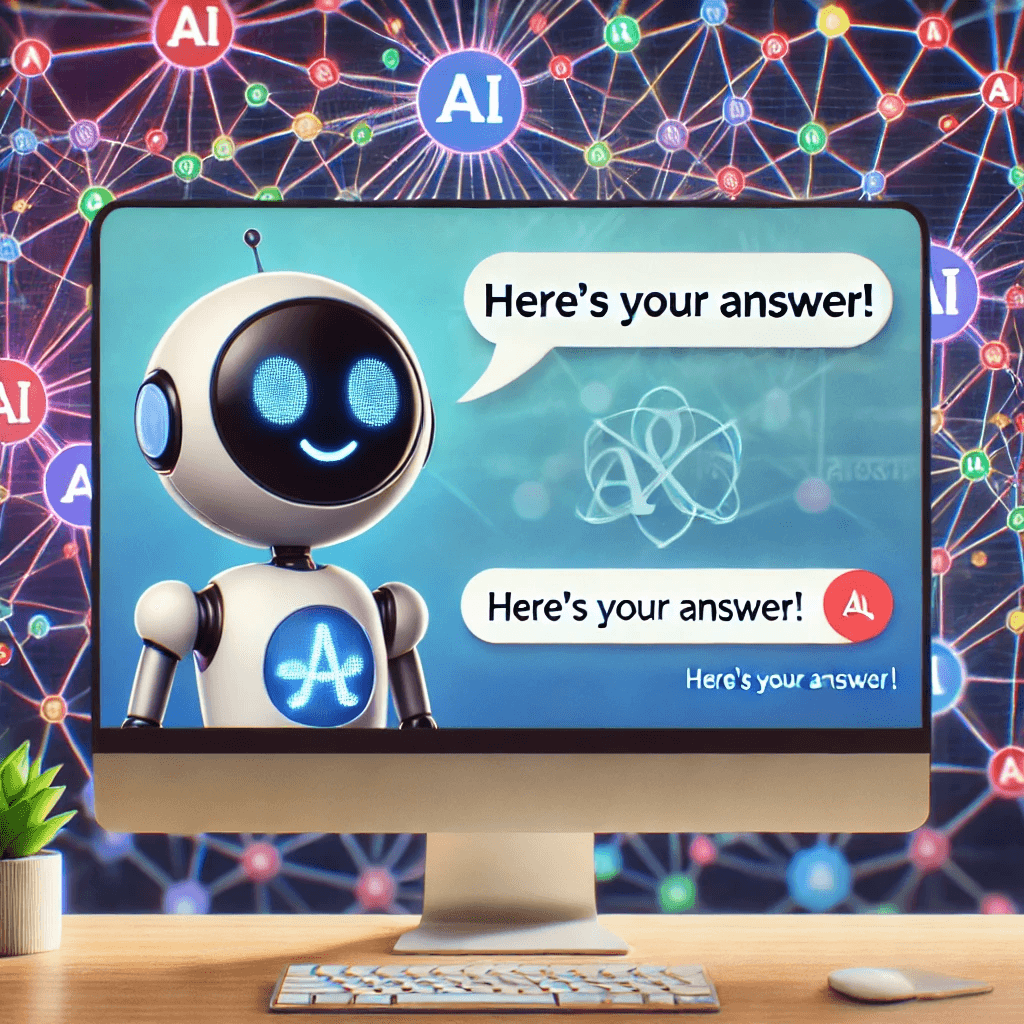
Today, LLMs are fundamentally changing how search engines function and how we search for information. Here’s how:
Contextual Understanding Beyond Keywords: Traditional search engines largely relied on keywords to deliver relevant results. LLMs, however, understand the context and intent behind search queries. They can process entire sentences and even paragraphs to understand user intent more deeply. This leads to more accurate and relevant results.
Conversational Search Experiences: One of the most significant changes LLMs bring is the shift towards conversational search. Unlike traditional search engines that return a list of links, AI-powered search models like ChatGPT or Bing Chat provide direct answers and can engage in multi-turn conversations. Users can ask follow-up questions, refining their search and obtaining more precise answers without needing to rephrase or type additional queries.
Answer Generation and Summarization: LLMs are capable of generating direct answers rather than merely linking to web pages. For example, if you ask an LLM-powered search engine, "What is quantum computing?" it can generate a concise yet comprehensive answer, pulling from a vast range of sources, instead of providing a list of links. This is particularly useful for users who want quick answers without diving deep into lengthy articles.
Personalized Search Results: With LLMs, search engines are better at personalizing results based on a user’s search history, preferences, and even geographical location. While traditional algorithms used rudimentary methods to personalize, LLMs can deliver content that aligns more closely with user behavior and interests by understanding context at a deeper level.
3. Benefits of LLM-Driven Search Engines
The integration of LLMs into search engines brings several advantages:
Enhanced User Experience: With conversational abilities and contextual understanding, users get more precise and relevant answers, which improves overall satisfaction.
Improved Accessibility: Users who may have difficulty forming precise queries can benefit from LLMs’ ability to understand vague or poorly formed questions and still provide useful results.
Content Discovery: LLMs can better surface high-quality, niche, or long-tail content that might not have ranked highly in traditional keyword-based searches.
4. Challenges and Limitations of LLM-Based Search
While LLMs enhance search engines significantly, they also introduce new challenges:
Accuracy and Trustworthiness: LLMs can generate answers that sound plausible but may be incorrect or misleading. Unlike traditional search engines that present sources, LLM-generated answers may not always be transparent about where the information comes from.
Bias and Fairness: LLMs can reflect biases present in the data they were trained on. This can lead to skewed results or answers that favor certain perspectives over others.
Privacy Concerns: Personalized search results powered by LLMs require more data about user behavior and preferences, raising privacy concerns.
5. The Future of Search with LLMs: What’s Next?
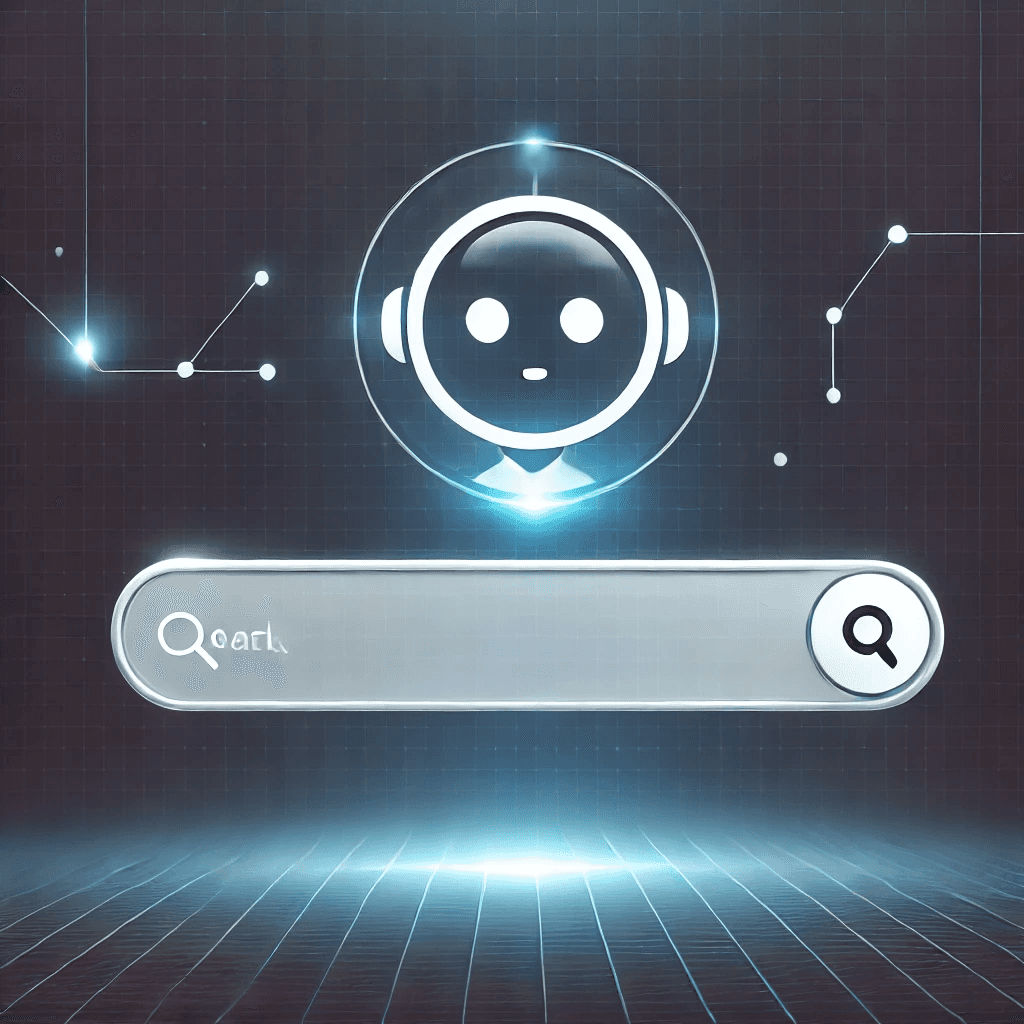
As LLMs continue to evolve, the future of search engines is poised for even more transformative changes:
Hybrid Models Combining LLMs and Traditional Search: The future of search might combine the best of both worlds—using LLMs to provide instant answers and engage in conversational queries while also providing traditional search results for more in-depth exploration.
Integration with Other AI Tools: We may see tighter integration of LLM-powered search engines with other AI tools, such as virtual assistants, augmented reality, and voice search, creating a more seamless, interactive user experience.
Better Handling of Misinformation and Biases: Researchers and developers are working on ways to mitigate the biases of LLMs and ensure the information provided is accurate and trustworthy. This could involve better training data, improved algorithms for content verification, and more transparent systems.
Conclusion: A New Era of Information Search
The integration of Large Language Models into search engines marks a new era in how we find and interact with information. With their ability to understand context, engage in conversations, and generate precise answers, LLMs are making search engines smarter and more user-friendly. However, as with any technology, it’s essential to navigate the challenges and ensure that these advancements benefit all users ethically and equitably.
The way we search for information is changing rapidly, and LLMs are at the forefront of this transformation. As they continue to evolve, they promise a future where finding information is not just about typing in keywords but engaging in meaningful dialogues with AI.