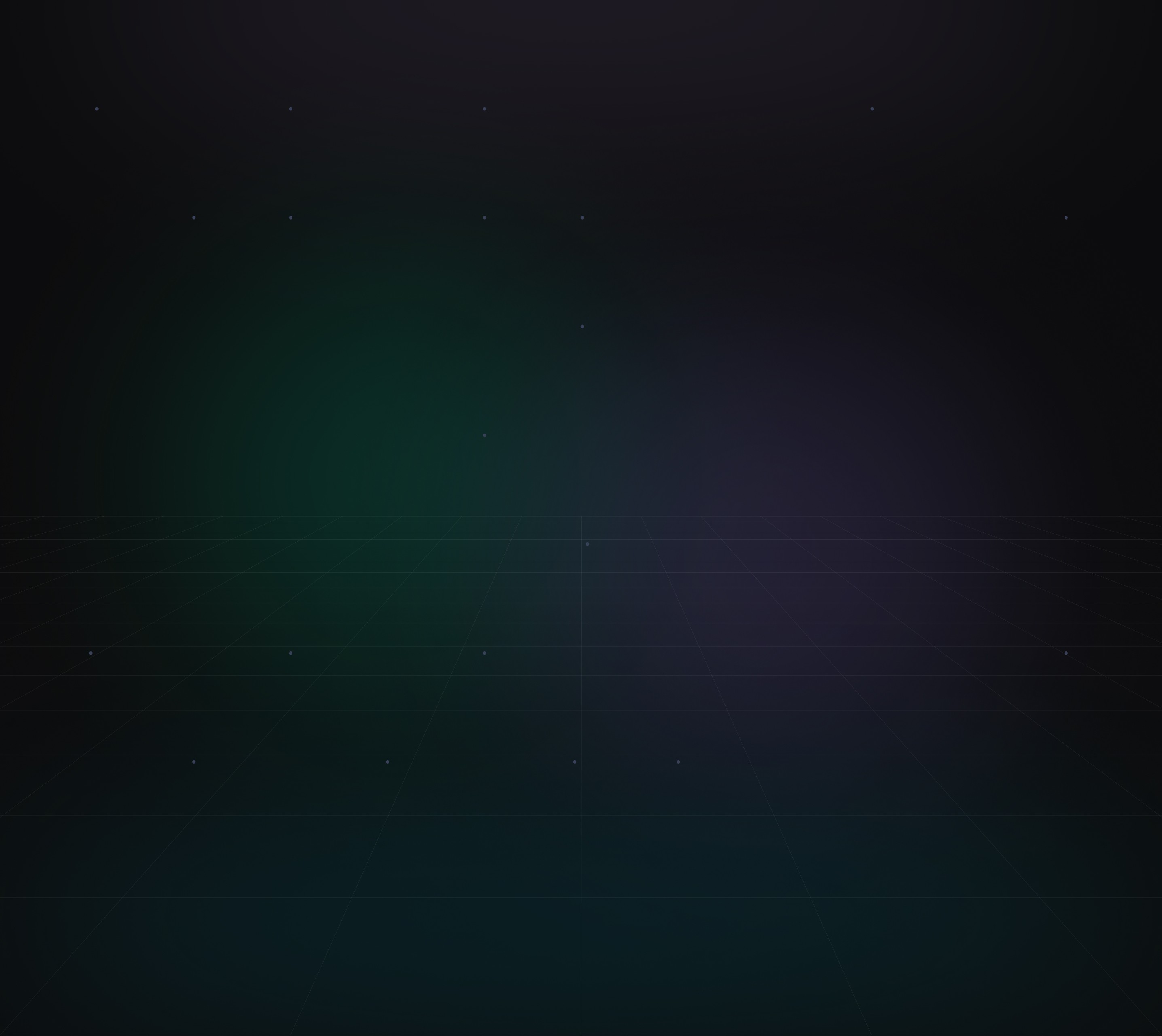

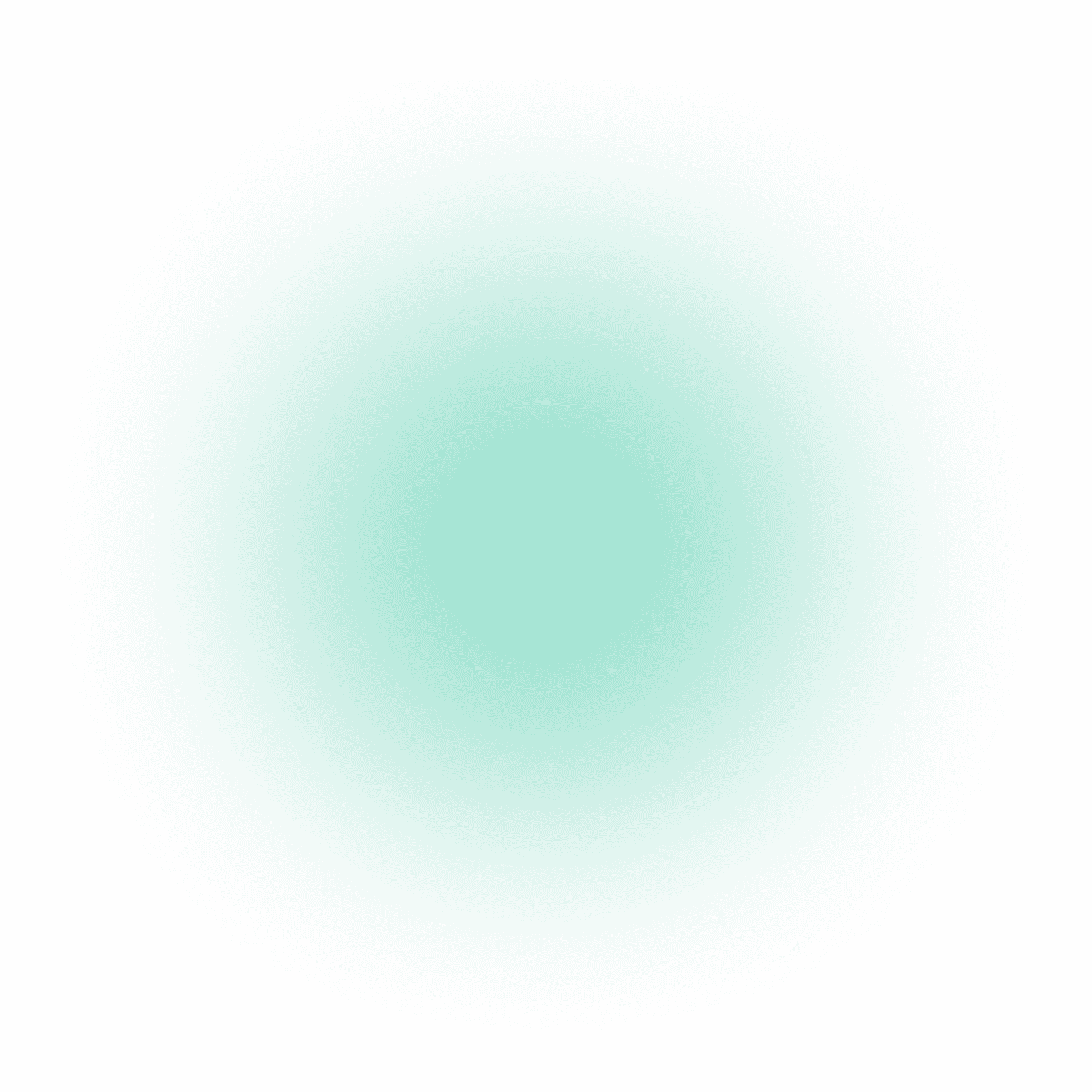

AI Decision-Making: Essential Insights for Managers Before Committing to LLM-Based Applications
Explore essential insights into AI decision-making that every manager must know. Learn about defining decision scopes, understanding AI biases, and implementing human oversight to responsibly integrate LLM-based applications.
Author
D Team
Aug 29, 2024
Artificial Intelligence (AI) is rapidly changing the landscape of business, promising efficiency, insights, and automation. Among the most impactful of these innovations are Large Language Models (LLMs), which power AI agents capable of making decisions, generating content, and interacting with customers. But as AI moves from buzzword to business tool, managers must understand the intricacies of AI decision-making before committing to these technologies. This guide aims to demystify AI decision-making, highlighting the key considerations, challenges, and best practices that every manager should know before integrating LLM-based applications into their workflow.
Understanding AI Decision-Making: Beyond the Hype
AI decision-making involves algorithms processing data, identifying patterns, and making predictions or recommendations based on that information. However, it’s crucial to understand that AI, especially LLMs, doesn’t think like humans. These systems are sophisticated pattern recognizers, not cognitive beings, and their “decisions” are outputs based on mathematical models trained on vast datasets.
Analogy: The Recipe Book Chef
Imagine AI as a chef following a recipe book with thousands of recipes but no personal taste or creativity. The chef selects recipes based on ingredients (data) and preferences (training), but they don’t truly understand what makes a dish delicious—they’re just following instructions. Similarly, LLMs make decisions based on statistical patterns, not human intuition or understanding.
Key Considerations for Managers Before Adopting LLM-Based Applications
1. Define the Decision-Making Scope Clearly
One of the first steps in adopting AI is defining the scope of decisions that AI will handle. Not all decisions are suitable for AI; the tasks you delegate to LLMs should be well-defined, repetitive, and data-driven.
Key Questions to Ask:
What types of decisions will the AI be making? (e.g., customer service responses, product recommendations, content generation)
Are these decisions low-risk or high-risk?
How much variability and nuance are involved in these decisions?
Example: Customer Support Automation If you’re using an AI to automate customer support, defining the decision scope is crucial. The AI can handle repetitive, straightforward inquiries (like FAQs), but complex or emotionally charged issues should be escalated to human agents. Understanding the limits of AI decision-making ensures the right balance between automation and human oversight.
2. Assess Data Quality and Availability
LLMs rely heavily on data to function effectively. Before implementing an AI system, assess the quality, relevance, and availability of your data. Poor-quality data can lead to biased, inaccurate, or unreliable AI decisions.
Data Assessment Checklist:
Data relevance: Is the data applicable to the decisions the AI needs to make?
Data quality: Is the data clean, well-structured, and up-to-date?
Data volume: Is there enough data to train the AI model effectively?
Analogy: Garbage In, Garbage Out (GIGO) Think of AI as a powerful sports car—it performs brilliantly only with high-quality fuel. In this case, data is the fuel. Feeding your AI with biased, incomplete, or irrelevant data will result in poor performance, much like running a high-performance car on low-quality fuel.
3. Understand the Limitations and Biases of LLMs
LLMs are trained on vast amounts of text data, which means they can reflect biases present in that data. Understanding these biases and limitations is crucial for managers to set realistic expectations and implement mitigation strategies.
Common Limitations of LLMs:
Bias Reflection: LLMs can replicate biases found in their training data, affecting decisions related to gender, race, and other sensitive topics.
Lack of Contextual Understanding: AI can misinterpret nuanced or ambiguous inputs, leading to inappropriate or incorrect decisions.
Data Sensitivity: LLMs cannot access or learn from new data unless retrained, limiting their ability to adapt in real-time.
Example: Content Moderation If you’re using an LLM for content moderation, be aware that the AI might flag content incorrectly due to biases in training data. For instance, certain phrases or tones might be misinterpreted, leading to unnecessary censorship or failure to catch harmful content. Regularly reviewing and adjusting the AI’s decision-making criteria is essential to ensure accuracy.
4. Set Up Human-in-the-Loop Mechanisms
AI decision-making is powerful, but it’s not infallible. Setting up a human-in-the-loop (HITL) approach ensures that critical decisions have human oversight, reducing the risk of errors and enhancing accountability.
Benefits of HITL:
Error Correction: Humans can catch mistakes that AI might miss.
Ethical Oversight: Humans can provide ethical considerations that AI cannot.
Continuous Improvement: Feedback loops from human reviews help refine AI models.
Practical Application: Financial Services In financial services, AI can analyze data and make recommendations for investments, but human experts review these recommendations before execution. This approach leverages AI’s speed and analytical power while ensuring that the final decisions align with ethical standards and human judgment.
5. Evaluate the Impact of AI on Workflow and Staff
Integrating AI into decision-making processes can significantly impact your existing workflows and staff roles. It’s crucial to plan for these changes to ensure a smooth transition.
Key Impacts to Consider:
Role Adjustments: Staff may need to shift from doing tasks to overseeing AI or managing exceptions.
Training Needs: Employees may require training to work effectively alongside AI systems.
Change Management: Communicate clearly with staff about how AI will affect their roles to minimize resistance and maximize buy-in.
Example: Implementing AI in HR If an AI system is used for initial resume screening, HR professionals might shift their focus to more strategic tasks like interviews and candidate relationship management. Preparing your team for these changes is crucial to maintaining morale and ensuring the successful adoption of AI.
Best Practices for Implementing AI Decision-Making in Business
1. Pilot Small, Scale Gradually
Start with a pilot project that involves a specific, low-risk decision-making process. This approach allows you to test the AI’s capabilities, gather data, and make adjustments before scaling up.
Example: AI for Customer Feedback Analysis Instead of deploying AI across all customer service channels, start by using it to analyze customer feedback from one platform, such as email. Monitor the AI’s performance, adjust parameters as needed, and gradually extend its use to other areas.
2. Regularly Review and Update AI Models
AI decision-making models require regular reviews to ensure they remain accurate, relevant, and unbiased. As business needs evolve, so should your AI systems.
Practical Tip: Establish a schedule for reviewing AI models, including checking decision accuracy, updating training data, and retraining the model if necessary. Incorporate feedback from human reviewers to fine-tune the AI’s performance.
3. Prioritize Transparency and Explainability
For managers, understanding how AI makes decisions is crucial to trust and accountability. LLMs are often seen as “black boxes,” where the decision-making process is opaque. Investing in explainable AI (XAI) tools can help clarify how decisions are made, providing insights into the logic behind AI outputs.
Example: Explainable AI in Healthcare In healthcare, AI systems used for diagnostic support must provide clear explanations of their recommendations to ensure doctors understand and trust the outputs. Implementing XAI can help bridge the gap between AI predictions and human decision-making.
4. Establish Clear Governance and Accountability
Implementing AI decision-making requires clear governance structures to manage accountability. This includes defining who is responsible for overseeing AI decisions, how risks will be managed, and what protocols are in place for handling errors.
Governance Tips:
Define roles and responsibilities for AI oversight.
Create a risk management framework specific to AI-related decisions.
Document decision-making processes to ensure traceability and accountability.
Conclusion: Informed AI Adoption Leads to Better Business Outcomes
AI decision-making offers enormous potential, but it’s essential for managers to approach it with a clear understanding of its capabilities, limitations, and implications. By defining decision scopes, assessing data quality, integrating human oversight, and establishing robust governance, managers can harness the power of LLM-based applications responsibly and effectively. Remember, the goal is not just to automate decisions but to enhance them—combining the strengths of AI with the irreplaceable judgment of human expertise.